Table of Contents
Most people like





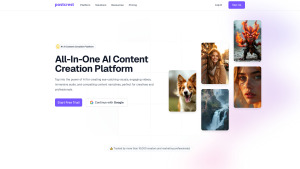

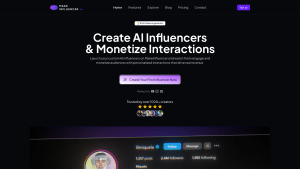

- App rating
- 4.9
- AI Tools
- 100k+
- Trusted Users
- 5000+
TOOLIFY is the best ai tool source.
- Unlock the Secret of Image Annotation and Earn Money Now
- Unlocking the Power of Employee Feedback
- Unveiling IA-Cloud: A Revolutionary Perspective by UiQ
- Unlocking the Power of Embedded AI Systems and Edge Computing
- Where to Trade Cryptocurrency: Best Platforms for Beginners and Experienced Traders
- Top 7 Cheapest Crypto Trading Platforms for Low-Fee Trading in 2023
- Top 3 Low-Fee Crypto Trading Exchanges to Maximise Your Profits in 2023
- Best Leverage Trading Platforms for Crypto: Top Choices for Safe and Profitable Trading
- Best Futures Trading Platforms for Crypto in 2023: Top Choices for Beginners & Experts
- How to Choose the Right Video Editor for Your Projects
- Transform Your Images with Microsoft's BING and DALL-E 3
- Create Stunning Images with AI for Free!
- Unleash Your Creativity with Microsoft Bing AI Image Creator
- Create Unlimited AI Images for Free!
- Discover the Amazing Microsoft Bing Image Creator
- Create Stunning Images with Microsoft Image Creator
- AI Showdown: Stable Diffusion vs Dall E vs Bing Image Creator
- Create Stunning Images with Free Ai Text to Image Tool
- Unleashing Generative AI: Exploring Opportunities in QE&T
- Create a YouTube Channel with AI: ChatGPT, Bing Image Maker, Canva
- Google's AI Demo Scandal Sparks Stock Plunge
- Unveiling the Yoga Master: the Life of Tirumalai Krishnamacharya
- Hilarious Encounter: Jimmy's Unforgettable Moment with Robert Irwin
- Google's Incredible Gemini Demo: Unveiling the Future
- Say Goodbye to Under Eye Dark Circles - Simple Makeup Tips
- Discover Your Magical Soul Mate in ASMR Cosplay Role Play
- Boost Kidney Health with these Top Foods
- OpenAI's GEMINI 1.0 Under Scrutiny
- Unveiling the Mind-Blowing Gemini Ultra!
- Shocking AI News: Google's Deception Exposed!
- Exploring Minecraft 19.2: Performance, Settings, and Skyward Adventure
- Unleash the Power: Building a Gaming PC with Server Gear
- How to Setup Xbox Game Pass Cloud Gaming on Android TV
- Unlocking the Full Potential of AMD 1055T: Overclocking Adventure
- Performance Test: 4 Two-in-One Devices Compared
- Gaming on an Nvidia Quadro Card: Can It Deliver a Satisfying Experience?
- Intel's New Core i9-14900K: Faster than Core i9-13900K?
- Unleashing the Power: Ryzen 7 1700 vs 2700X Performance Comparison
- Essential Hardware and Software for Starting a Business
- Want to enhance your VR headset experience with AI? Here's how to do it!