Most people like
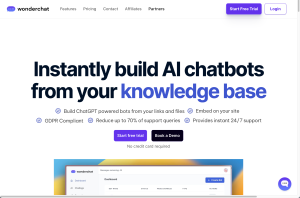




























- App rating
- 4.9
- AI Tools
- 100k+
- Trusted Users
- 5000+
TOOLIFY is the best ai tool source.
- How AI Tools Can Simplify Diet Planning
- 5 Types of Marketing to Boost Your Business Results in 2024
- How RedSky e911 Improves Emergency Communication and Response
- Top Tools for Streamlining Your Business Processes
- The 5 Best Free AI Girlfriend Apps of 2024
- 7 Creative Uses for AI Tools in Everyday Life
- The Future of Smart Shopping: How AI & Cashback Apps Are Enhancing the Consumer Experience
- Rise of Instant Payments for Business
- Fotor Review for 2024 - The Online Photo Editor You Must Try Out (Exclusive Discount Code)
- 39+ AI tools for becoming better at Marketing
- Transform Your Images with Microsoft's BING and DALL-E 3
- Create Stunning Images with AI for Free!
- Unleash Your Creativity with Microsoft Bing AI Image Creator
- Create Unlimited AI Images for Free!
- Discover the Amazing Microsoft Bing Image Creator
- Create Stunning Images with Microsoft Image Creator
- AI Showdown: Stable Diffusion vs Dall E vs Bing Image Creator
- Create Stunning Images with Free Ai Text to Image Tool
- Unleashing Generative AI: Exploring Opportunities in QE&T
- Create a YouTube Channel with AI: ChatGPT, Bing Image Maker, Canva
- Google's AI Demo Scandal Sparks Stock Plunge
- Unveiling the Yoga Master: the Life of Tirumalai Krishnamacharya
- Hilarious Encounter: Jimmy's Unforgettable Moment with Robert Irwin
- Google's Incredible Gemini Demo: Unveiling the Future
- Say Goodbye to Under Eye Dark Circles - Simple Makeup Tips
- Discover Your Magical Soul Mate in ASMR Cosplay Role Play
- Boost Kidney Health with these Top Foods
- OpenAI's GEMINI 1.0 Under Scrutiny
- Unveiling the Mind-Blowing Gemini Ultra!
- Shocking AI News: Google's Deception Exposed!
- Can AMD's FSR Save Nvidia GT 1030? Review & Benchmark
- Experience the Power of Dell Precision 5530: 4K Display, NVIDIA Quadro, and More!
- Optimize Mining Performance with AMD & NVIDIA Mixed Card in HIVEOS
- Unleash the Power: Building a Gaming PC with Server Gear
- How to Setup Xbox Game Pass Cloud Gaming on Android TV
- Unlocking the Full Potential of AMD 1055T: Overclocking Adventure
- Performance Test: 4 Two-in-One Devices Compared
- Gaming on an Nvidia Quadro Card: Can It Deliver a Satisfying Experience?
- Intel's New Core i9-14900K: Faster than Core i9-13900K?
- Unleashing the Power: Ryzen 7 1700 vs 2700X Performance Comparison